Traditionally, the oil and gas (O&G) industry has been anchored in time-honored practices and a cautious approach. However, the tide is shifting as artificial intelligence (AI) begins to carve out its niche in this sector.
A significant portion of industry leaders—50% of those surveyed—report that they have already implemented AI to tackle various organizational challenges. This trend is not surprising. AI offers a range of benefits from the initial stages of exploration to the final phases of distribution. It enhances efficiency, reduces operational costs, and bolsters safety measures.
But how exactly is AI propelling the growth agenda of O&G companies?
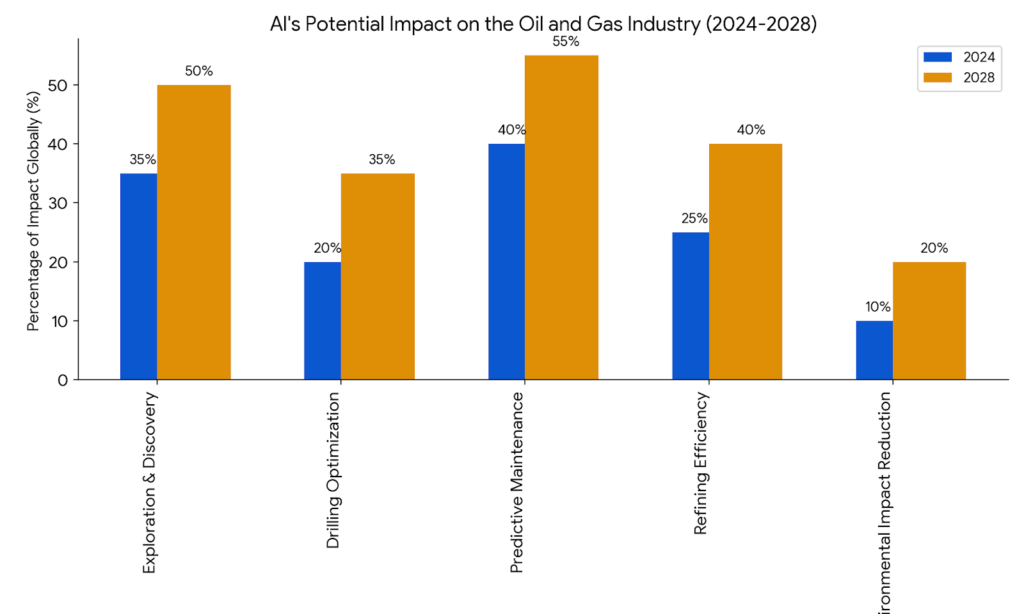
As the O&G sector evolves with AI-driven solutions, one particular branch—Generative AI—stands out for its ability to create transformative content and insights, paving the way for unprecedented advancements. Let’s explore what Generative AI is and a comprehensive transformation of Gen AI in the Oil and Gas Industry.
What is Generative AI?
Generative AI is a specialized area within artificial intelligence focused on creating new content such as images, videos, or music based on user instructions, known as “prompts.” This innovative technology leverages extensive data from various web platforms like Google, Facebook, and X (formerly known as Twitter) to produce these creative outputs.
Generative AI is revolutionizing how professionals handle tasks, analyze data, and communicate complex ideas in the oil and gas industry.
How Does Generative AI Work?
Generative AI follows a structured process to produce content based on user prompts:
- User Input: Users provide specific prompts or instructions to guide the AI in generating the desired output.
- Data Analysis: The AI analyzes vast amounts of data from diverse sources across the internet.
- Algorithmic Processing: Sophisticated algorithms process the input and data, identifying patterns and creating new content.
- Creation of Output: Based on the learned patterns and user prompts, the AI generates new images, videos, or music.
- Feedback Loop: Users offer feedback on the generated content, helping to refine and improve the AI’s performance over time.
This iterative process ensures that Generative AI continually enhances its ability to produce a wide range of meaningful and diverse content.
Oil and Gas Industry with Gen AI: A Comprehensive Transformation
Upstream | Midstream | Downstream | Cross-stream |
Exploration and Production Reservoir prediction and analysis Drilling operations Equipment maintenance Field worker safety with AI assistants | Storage and Transportation Inspecting storage facilities Logistics routes optimization | Refinery and distribution Refinery process optimization Quality standardization Product research and development | Asset maintenance planning Supply chain operations automation |
AI is revolutionizing the oil and gas industry by integrating into every phase of the supply chain. This technological advancement propels companies towards sustainable development and achieving net zero goals.
Upstream Use Cases: Exploration and Production
Precise Reservoir Prediction and Analysis
Traditional Methods
- Reservoir engineers sift through extensive electromagnetic and seismic data to locate new hydrocarbon deposits.
- This conventional method is costly, risky, and susceptible to errors.
- Mistakes can result in drilling wells in unproductive spots, each costing between $5 to $20 million.
AI Enhancements
- AI significantly mitigates these risks by enhancing the interpretation of seismic images.
- Geoscientists can use AI to:
- Filter out noise
- Improve image resolution
- Detect subtle geological features
- Generate additional data samples when the imagery is incomplete
AI-driven generative Models
- Analyze geological maps, production data, and well logs.
- Create detailed geo-models of hydrocarbon reservoirs.
- Aid engineers in predicting fluid movement and long-term well performance.
- Lead to more informed oil well placements.
Edge AI and IoT
- Enable real-time local processing of sensor data.
- Reduce the time to create geo-models from months to hours, as highlighted by Deloitte.
AI’s integration into reservoir prediction and analysis transforms the efficiency and accuracy of hydrocarbon exploration, ultimately reducing costs and increasing productivity.
Optimizing Drilling Operations
Given the high costs associated with drilling, oil and gas companies strive to optimize operations from the outset. Generative AI plays a key role in drilling optimization by transforming historical and real-time data into actionable insights. Advanced machine learning algorithms help steering teams analyze terabytes of historical data to determine optimal drilling parameters, such as weight on bit, speed, and angle. Neural networks use real-time drilling data to predict potential issues like stuck pipe events, allowing engineers to take proactive measures. This predictive intelligence reduces drill-bit failures and maximizes extraction rates.
Proactive Equipment Maintenance
Maintaining and Monitoring Equipment
For oil and gas producers, keeping exploration and production equipment in top shape is key.
Challenges with Manual Monitoring:
- Error-prone
- Time-consuming
- Ineffective use of historical data
AI-Driven Fault Detection
- Identifies patterns
- Predicts potential failures
- Optimizes maintenance schedules
Advanced Inspection Techniques
- Drones and Robots:
- Equipped with sensors and cameras
- Perform precise inspections
- Identify issues like cracks and corrosion
- Machine-Learning Models:
- Pre-trained to detect specific problems
- Enable continuous, real-time inspection
Benefits
- Minimizes operational risks
- Reduces expenses
Shell, for example, uses an ML-based predictive analytics solution to prevent critical equipment outages and determine when maintenance is needed, allowing their staff to focus on more strategic engineering tasks.
Enhancing Field Worker Safety with AI Assistants
Exploration environments are fraught with high pressures, heat, flammable substances, and other hazards that have historically led to safety incidents. AI-powered virtual assistants provide drilling rig crews, well operators, and technicians with quick and easy access to critical information. Integrated into field-friendly devices, these conversational AI assistants offer round-the-clock availability and are more effective in emergencies than human-staffed call centers.
Midstream Use Cases: Storage and Transportation
Here are some key use cases that midstream oil and gas leaders should be aware of:
Inspecting Storage Facilities
- Data Capture: Optical Gas Imaging (OGI) cameras mounted on robots or unmanned drones capture large volumes of data.
- Data Processing: Generative AI processes this data and provides natural language summaries.
- Efficiency: This technology drastically reduces the time previously spent on manual footage reviews.
- Safety: Operators can take necessary actions without entering hazardous areas, enhancing safety.
Optimizing Logistics Routes
- Data Analysis: Logistics specialists handle extensive data related to:
- Weather conditions
- Route hazards
- Port congestions
- Vessel conditions
- Other operational factors
- Advanced Analytics: Uncover insights to plan the most cost-effective routes for tankers.
- AI Optimization Algorithms: Ensure timely deliveries by:
- Identifying risks
- Adjusting routes in real-time without increasing the planned transit time.
Example: Shell’s LNG Shipping Accelerator consolidates critical infrastructure data for freight operators, reducing port waiting times and fuel consumption. This system ensures timely and environmentally friendly energy deliveries.
Downstream Use Cases: refinery and distribution
Downstream firms can reduce refinery and distribution costs while also achieving regulatory compliance faster by using AI.
Optimizing the refinery process
In downstream oil and gas firms, AI is used to optimize refineries through real-time monitoring systems. These systems keep track of activities and collect data during distillation, catalytic cracking, and hydrogenation. They also analyze data from energy meters and equipment sensors.
All of this adds to increased petrochemical throughput, reduced energy usage, and identification of potential safety concerns.
Meet quality standards faster
AI assists oil and gas refinery companies in meeting significant quality standards like ISO, API, ASTM, and others.
Straight from the production lines, ML algorithms, and predictive AI models assess the produced diesel, lubricants, jet fuel, natural gas, liquefied petroleum gas, oil petrochemicals, and so on to the specifications.
Production specialists can reduce waste and ensure production reliability and environmental sustainability by predicting fluctuations in product quality.
Product research and development
Generative AI in the oil and gas industry permits petrochemical engineers to speed materials development and save R&D costs by:
developing high-entropy alloys (HEAs) with superior mechanical, chemical, and physical properties; creating new chemical compounds with a variety of compositions; modeling these virtual assets’ behavior under various circumstances before actual physical production; and determining the most effective experimental protocols for material probing or optimization.
Cross-stream Use Cases
Some AI use cases in oil and gas are so diverse that they encompass numerous segments and operate across multiple layers of the industry.
Proactive asset maintenance planning
AI-powered predictive maintenance extends beyond upstream.
It predicts failures in pipelines, pump stations, and processing plant equipment to avoid costly repairs. Predictive maintenance models effectively maximize efficiency and prolong the lifespan of infrastructure throughout the whole oil and gas supply chain by maintaining a broad reach.
Furthermore, gen AI generates efficient maintenance schedules by analyzing data such as equipment usage, production needs, and required expenses.
Automating key supply chain operations
Apart from optimizing costly transits of crude oil or LNG via barges and tankers, advanced analytics algorithms improve the following:
Other transportation options include pipelines, trucks, and railroads. The distribution network layout includes storage facility locations, transit routes, and inventory levels.
Oil and gas firms and logistics providers use Gen AI to automate mission-critical supply chain procedures.
- Procurement involves anticipating material demand and choosing the best suppliers.
- On-shore and off-shore inventory management enhances asset tracking.
- Route planning includes analyzing current traffic conditions, optimizing ideal delivery timing, tracking vehicles, and fuel-efficient routes.
- Contingency planning entails running what-if scenarios in a digital twin environment to create tailored multi-purpose mitigation measures.
Segment | Use Case | Impact | Technologies Involved |
Upstream | Precise Reservoir Prediction | Reduces drilling risks and optimizes oil well placement. | Generative AI, Machine Learning, Edge AI, IoT |
Optimizing Drilling Operations | Reduces drill-bit failures, maximizes extraction rates, and avoids costly mistakes. | Generative AI, Machine Learning, Neural Networks | |
Proactive Equipment Maintenance | Minimizes operational risks, reduces expenses, and prevents critical equipment outages. | Machine Learning, Drones, Robots | |
Enhancing Field Worker Safety | Improves response time in emergencies and reduces safety incidents. | Conversational AI | |
Midstream | Inspecting Storage Facilities | Improves operational efficiency, and enhances safety by keeping workers out of hazardous areas. | Generative AI, Optical Gas Imaging (OGI) |
Optimizing Logistics Routes | Ensures timely deliveries, reduces fuel consumption and improves environmental impact. | Advanced Analytics, AI Optimization Algorithms | |
Downstream | Optimizing Refinery Process | Increases throughput, reduces energy usage, and improves safety. | Real-time monitoring systems, Machine Learning |
Meeting Quality Standards | Reduces waste, ensures production reliability, and promotes environmental sustainability. | Machine Learning, Predictive AI Models | |
Product Research and Development | Speeds up materials development reduces R&D costs, and leads to innovative products. | Generative AI | |
Cross-stream | Proactive Asset Maintenance | Maximizes efficiency, prolongs the lifespan of infrastructure and avoids costly repairs. | Predictive Maintenance Models, Generative AI |
Automating Key Supply Chain Operations | Improves efficiency, reduces costs, and optimizes the entire oil and gas supply chain. | Advanced Analytics Algorithms, Generative AI |
Five Principles of Generative AI in the Oil and Gas Industry
Generative AI is revolutionizing the oil and gas industry by enhancing operational efficiency, reducing costs, and driving profitability. Here are five core principles guiding its implementation:
1. Enhanced Exploration and Production
Maximizing Existing Capabilities with Gen AI
Generative AI enhances existing exploration and production (E&P) initiatives. By integrating AI with current data and models, companies can make better, faster decisions. For example, AI can analyze seismic data, predict reservoir locations, and optimize drilling operations, leading to more efficient resource extraction and reduced environmental impact.
Real-world Application: AI-driven predictive models can anticipate equipment failures, allowing for timely maintenance and reducing downtime, which is critical in the high-stakes world of oil and gas.
2. Optimized IT Infrastructure
Building on Existing Digital Maturity
Gen AI thrives on the foundation of an organization’s existing IT infrastructure. It’s essential to start with use cases that align with current digital capabilities, enabling a gradual, integrated approach to technology adoption. This ensures the IT architecture evolves alongside AI advancements.
Consideration: When deploying AI, it’s vital to assess its impact on IT systems, ensuring scalability and compatibility with existing and future digital ecosystems.
3. Workforce Training and Process Integration
Empowering Employees with AI Knowledge
While AI automates many tasks, human expertise remains indispensable. Training programs must evolve to cover AI tools’ functionalities and limitations, ensuring employees can effectively collaborate with AI and fill in the gaps where needed.
Example: In the context of safety management, AI can predict potential hazards, but workers need to interpret these predictions and take appropriate actions, combining AI insights with their on-the-ground experience.
4. Comprehensive Risk and Change Management
Ensuring Robust Data Security and Compliance
Data is the backbone of AI, making risk management paramount. This includes safeguarding sensitive information and addressing operational, data, and legal risks. Establishing a robust risk management framework at the outset of AI programs is essential.
Strategy: Implementing stringent security protocols and continuously updating risk management strategies can help mitigate potential threats, ensuring compliance with evolving regulations.
5. Financials and Performance Metrics
Maximizing ROI through Outcome Management
Building an extensive outcome management framework is essential for realizing the full potential of generation AI. This includes defining clear metrics and benchmarks to measure the effectiveness and ROI of AI implementations.
Key Element: Utilizing techniques like A/B testing to compare AI-enhanced processes with traditional methods helps quantify efficiency gains and identify areas for improvement.
By adhering to these principles, the oil and gas industry can effectively leverage generative AI to transform operations, drive innovation, and achieve sustainable growth.
Accelerating Innovation with Generative AI in the Oil and Gas Sector
The oil and gas industry is in a constant state of flux, requiring companies to innovate continuously to maintain a competitive edge.
Generative AI is emerging as a crucial tool for fostering innovation and speeding up the development of new technologies, processes, and solutions to tackle the industry’s most significant challenges.
By harnessing AI-driven insights and simulations, companies can rapidly explore novel ideas and approaches, identifying the most promising opportunities and fine-tuning them to meet specific needs and constraints.
This accelerated exploration can lead to groundbreaking solutions that have the potential to revolutionize the industry, paving the way for a more sustainable and technologically advanced future for oil and gas companies in the digital era.
Conclusion
Generative AI is set to transform the oil and gas industry, enhancing efficiency, safety, and sustainability across all value chain stages.
By leveraging AI, companies can optimize exploration activities, boost production efficiency, enhance safety and risk management, streamline transportation and distribution, and promote supply chain sustainability.
As generative AI technologies continue to evolve, their applications and impact on the oil and gas sector will grow even more significant.
Companies that embrace and adapt to these emerging technologies will be well-positioned to seize their numerous opportunities, ensuring a prosperous future in the global energy market.